Importance Score: 45 / 100 🔵
AI Benchmark Debate Extends to Pokémon Comparisons
Even the realm of Pokémon is not immune to the ongoing discussions surrounding artificial intelligence benchmarking. Recent social media buzz highlighted claims that Google’s Gemini model outperformed Anthropic’s Claude in navigating the original Pokémon video games. This assertion, suggesting Gemini progressed further in the game, ignited discourse on the validity and interpretation of AI model evaluations.
Initial Claims Sparked Online Discussion
Last week, a post on the social media platform X gained traction, asserting that Google’s advanced Gemini model demonstrated superior performance compared to Anthropic’s Claude in the context of the classic Pokémon game trilogy. The post indicated that during a developer’s live stream on Twitch, Gemini had reached Lavender Town, while Claude was reportedly still navigating Mount Moon as of late February.
Contextual Factors in Pokémon Benchmark Results
However, the viral post omitted crucial context regarding the testing methodologies.
Gemini’s Minimap Advantage
As Reddit users quickly pointed out, the developer overseeing the Gemini stream incorporated a bespoke minimap feature. This custom tool aided the AI model in identifying in-game “tiles,” such as trees that could be cut down. This supplemental information reduced Gemini’s reliance on solely analyzing visual screenshots to formulate gameplay decisions, providing a distinct advantage.
The Nuances of AI Benchmarking
While Pokémon may be considered a lighthearted or semi-serious AI benchmark, its use highlights a significant issue: the informative value of such tests in accurately representing a model’s capabilities is debatable. Nevertheless, this example effectively illustrates how variations in benchmark implementation can significantly skew results and complicate direct model comparisons.
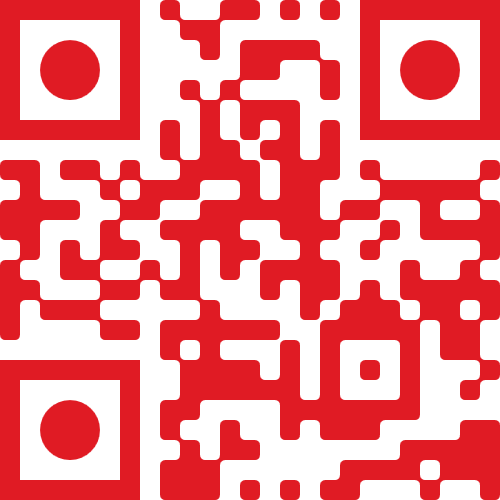
vCard.red is a free platform for creating a mobile-friendly digital business cards. You can easily create a vCard and generate a QR code for it, allowing others to scan and save your contact details instantly.
The platform allows you to display contact information, social media links, services, and products all in one shareable link. Optional features include appointment scheduling, WhatsApp-based storefronts, media galleries, and custom design options.
Benchmark Implementation and Performance Variation
Illustrating this point, Anthropic previously disclosed two distinct performance metrics for their Anthropic 3.7 Sonnet model on the SWE-bench Verified benchmark, designed to evaluate coding proficiency. Claude 3.7 Sonnet achieved a 62.3% accuracy score on SWE-bench Verified under standard conditions. However, when utilizing a “custom scaffold” developed by Anthropic, the model’s accuracy improved to 70.3%.
In a more recent instance, Meta refined a version of its Llama 4 Maverick model to specifically excel on the LM Arena benchmark. The standard, unmodified version of this model performs considerably worse on the same evaluation, underscoring the impact of targeted optimization on benchmark outcomes.
Challenges in Model Comparison
Considering the inherent limitations of AI benchmarks, including unconventional examples like Pokémon, the introduction of customized and non-standard implementations further obscures meaningful comparisons between different AI models. Consequently, as new models are released, accurately assessing and contrasting their relative performance is likely to become increasingly complex and challenging.