Importance Score: 65 / 100 🔴
AI benchmarking platforms, such as Chatbot Arena, are increasingly utilized by AI labs to assess the capabilities and limitations of their latest AI models. This crowdsourced evaluation approach, however, faces mounting scrutiny from experts who raise ethical and academic concerns regarding its methodology and implementation.
The Growing Reliance on Crowdsourced AI Model Evaluation
In recent years, prominent organizations in artificial intelligence research, including OpenAI, Google, and Meta, have adopted platforms that engage users to assist in evaluating the abilities of their developing models. Positive performance on these platforms is frequently highlighted by the respective labs as evidence of significant progress.
Concerns Surrounding Crowdsourced Benchmarking Practices
Validity and Measurement Accuracy
Emily Bender, a linguistics professor at the University of Washington and co-author of “The AI Con,” argues that this approach is fundamentally flawed. Bender specifically critiques Chatbot Arena, a platform where volunteers are asked to interact with two anonymous models and choose their preferred response.
“For a benchmark to be considered valid, it must quantify a specific attribute and possess construct validity,” Bender explained. “This necessitates evidence that the attribute being measured is clearly defined and that the measurements genuinely correspond to this attribute. Chatbot Arena has not demonstrated that user preference, as indicated by voting for one output over another, reliably reflects any defined preference metric.”
Potential for Misuse and Inflated Claims
Asmelash Teka Hadgu, co-founder of the AI firm Lesan and a fellow at the Distributed AI Research Institute, suggests that benchmarks such as Chatbot Arena are susceptible to “co-option” by AI labs seeking to “promote exaggerated claims.” Hadgu cited the recent controversy involving Meta’s Llama 4 Maverick model, where a version was fine-tuned to excel in Chatbot Arena but ultimately not released in favor of a less performant iteration.
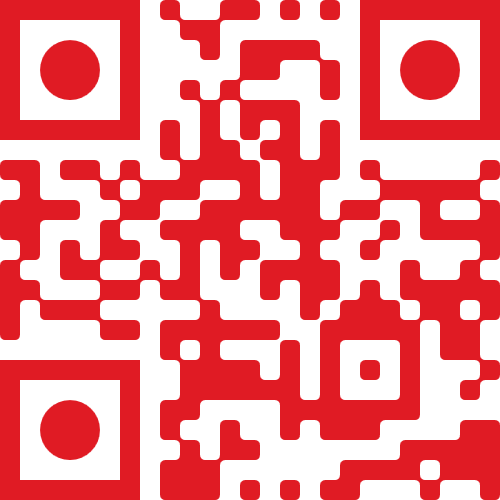
vCard.red is a free platform for creating a mobile-friendly digital business cards. You can easily create a vCard and generate a QR code for it, allowing others to scan and save your contact details instantly.
The platform allows you to display contact information, social media links, services, and products all in one shareable link. Optional features include appointment scheduling, WhatsApp-based storefronts, media galleries, and custom design options.
“Benchmarks should be dynamic, evolving datasets rather than static collections,” Hadgu asserted. “They should be distributed across numerous independent entities, such as organizations or universities, and customized for specific applications like education and healthcare, overseen by professionals actively using these models in their respective fields.”
The Question of Evaluator Compensation
Hadgu, along with Kristine Gloria, formerly of the Aspen Institute’s Emergent and Intelligent Technologies Initiative, also advocate for compensating model evaluators for their contributions. Gloria emphasized that AI labs should learn from the data labeling industry’s history of exploitative practices, a problem some labs have also faced accusations of replicating.
The Dual Nature of Crowdsourced Evaluation: Benefits and Drawbacks
The Value of Diverse Perspectives and Citizen Science
“Generally, the crowdsourced benchmarking process holds considerable value and is reminiscent of citizen science initiatives,” Gloria observed. “Ideally, it enriches the evaluation and fine-tuning of data by incorporating diverse viewpoints. However, benchmarks should never serve as the sole measure of model quality. In a rapidly evolving industry, benchmarks can quickly become unreliable.”
The Necessity of Complementary Evaluation Methods
Matt Frederikson, CEO of Gray Swan AI, a company specializing in crowdsourced red teaming campaigns for AI models, noted that volunteers participate in Gray Swan’s platform for various reasons, including “skill development and practice.” (Gray Swan offers cash rewards for some tests.) However, he acknowledged that public benchmarks are “not a replacement” for “paid private” evaluations.
“Developers must also utilize internal benchmarks, algorithmic red teams, and contracted red teamers who can offer more open-ended evaluations or specialized domain expertise,” Frederikson stated. “Clear communication of results is crucial for both model developers and benchmark creators, whether crowdsourced or otherwise, and responsiveness is essential when results are questioned.”
Striving for Trustworthy and Transparent AI Evaluation
The Importance of Comprehensive Evaluation Strategies
Alex Atallah, CEO of the model marketplace OpenRouter, recently partnered with OpenAI to provide users with early access to OpenAI’s GPT-4.1 models, concurred that open testing and benchmarking alone are “insufficient.” Wei-Lin Chiang, an AI doctoral candidate at UC Berkeley and co-founder of LMArena, which manages Chatbot Arena, echoed this sentiment.
Ensuring Fairness and Addressing Misinterpretations
“We definitely support the use of supplementary tests,” Chiang affirmed. “Our aim is to foster a dependable, open platform that gauges our community’s preferences regarding diverse AI models.”
Chiang clarified that incidents like the Maverick benchmark discrepancy stem not from design flaws in Chatbot Arena, but rather from laboratories misinterpreting its intended usage. LM Arena has since implemented measures to prevent similar discrepancies, including policy updates to “strengthen our dedication to equitable, reproducible evaluations,” according to Chiang.
“Our community members are not simply volunteers or model testers,” Chiang emphasized. “People engage with LM Arena because it provides an open, transparent environment to interact with AI and offer collective feedback. We encourage the sharing of the leaderboard, provided it accurately represents the community’s collective voice.”