Importance Score: 72 / 100 🔴
The Tacoma Narrows Bridge, famously dubbed “Galloping Gertie” for its undulating movements, experienced a catastrophic collapse on November 7, 1940, shortly after its inauguration on July 1, 1940. In a now-infamous engineering failure, moderate winds on that fateful morning triggered a repeated twisting motion in the bridge structure. After approximately an hour of escalating torsion, the suspension bridge succumbed to structural stress and collapsed. This dramatic event underscored a critical flaw in initial engineering design assumptions.
The Tacoma Narrows Bridge Disaster: A Case Study in Engineering Assumptions
The Perils of Overlooked Wind Dynamics
During that era, many structural engineers operated under the presumption that wind forces could not induce vertical movement in bridges. This misconception, while seemingly obvious in hindsight, proved costly, resulting in financial repercussions equivalent to roughly $65 million in today’s currency, and tragically, the loss of a dog.
Subtle vertical oscillations initiated a twisting phenomenon in the Tacoma Narrows Bridge. Ultimately, the bridge underwent unanticipated torsional distortions that far exceeded design parameters. This extreme twisting subjected the structure to immense strain, culminating in the Tacoma Narrows Bridge collapse.
By disregarding potential vertical wind effects, engineers neglected to adequately assess aerodynamic flutter during the bridge’s design phase. This critical oversight in considering wind dynamics ultimately doomed the structure, highlighting the crucial role of comprehensive engineering models.
Engineering Models and Real-World Limitations
The Tacoma Narrows incident serves as a stark illustration of a fundamental lesson for engineering students: all engineering calculations rely on models. Safe and robust design necessitates that engineers thoroughly understand the underlying assumptions within their models and ensure structural integrity despite any inherent limitations.
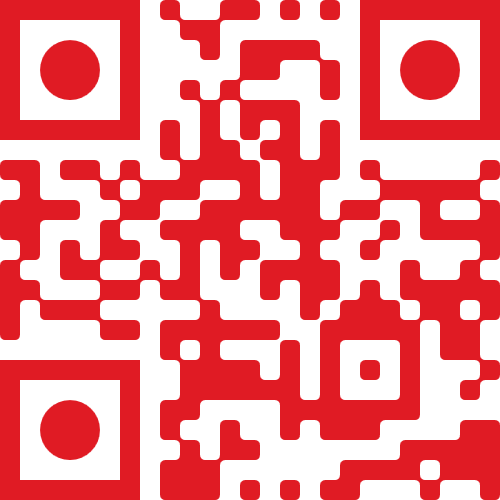
vCard.red is a free platform for creating a mobile-friendly digital business cards. You can easily create a vCard and generate a QR code for it, allowing others to scan and save your contact details instantly.
The platform allows you to display contact information, social media links, services, and products all in one shareable link. Optional features include appointment scheduling, WhatsApp-based storefronts, media galleries, and custom design options.
The careful application of models is paramount. As the renowned statistician George Box articulated, “All models are wrong – some are useful,” underscoring the balance between model utility and inherent inaccuracies.
Understanding and Utilizing Engineering Models
Models as Interpretive Frameworks
Engineering models function as interpretive frameworks, enabling scientists and engineers to correlate data with real-world phenomena. For example, a common understanding exists regarding material strength: excessive force applied to wood will cause it to fracture. A more resilient piece of wood can withstand greater force.
Engineers employ models to quantify this intuitive understanding with precision.
Engineering strength assessment utilizes an interpretive model that interrelates forces, object dimensions, and their ratio – mechanical stress. The engineering concept of “strength” is intrinsically linked to this calculated stress.
Stress analysis allows engineers to select materials with adequate strength for bridge construction and various other applications.
Simplifications and Assumptions in Modeling
However, all models inherently omit certain aspects of reality. Stress computation requires engineers to define an object’s geometry. Real-world objects possess complexity, necessitating shape simplification for computational purposes.
For instance, engineers might model a complex cable bundle as a uniform cylinder. This simplification assists in determining the number of wires required and the overall bundle diameter.
Assumptions introduce limitations: the cylinder simplification neglects individual wires and is inadequate for determining wire weaving patterns. While more detailed models can be employed when necessary, they too inevitably incorporate assumptions and constraints.
Balancing Assumptions and Limitations
This interplay between assumptions and limitations is fundamental to all models. The Tacoma Narrows Bridge engineers’ assumption of negligible wind-induced vertical movement led to a critical limitation: they could not foresee the wind-driven flutter that ultimately destroyed the bridge.
Analogous principles apply to abstract models, such as facial recognition systems based on artificial intelligence. The assumption of system accuracy based on training data performance can be misleading. External research has revealed limitations stemming from training dataset biases.
Datasets used to train these AI systems sometimes operate under the assumption of representative diversity but may underrepresent certain demographics, such as non-white individuals. This limitation has resulted in AI systems exhibiting disproportionate bias against specific populations.
In pursuit of enhanced AI, some researchers prioritize increased training data volume. However, this data-intensive strategy has environmental consequences due to the substantial energy consumption of large data centers.
Effective model utilization entails selecting assumptions whose limitations do not compromise the intended application. Rigorous testing is the ideal validation method, but practical constraints often preclude it – constructing a full-scale test bridge, for example, is infeasible.
Prudent model selection and development necessitate sound engineering judgment.
Cultivating Engineering Judgment Through Modeling Education
Developing Critical Evaluation Skills
Engineering judgment involves carefully balancing reliance on and skepticism towards mathematical frameworks that underpin many engineering models. Developing this essential judgment is a complex process typically cultivated through extensive practical experience. Educational initiatives, such as modeling and simulation courses, can accelerate the development of students’ engineering acumen.
Educational approaches that encourage students to construct their own models—an atypical experience in engineering curricula—can be particularly valuable. Students can then identify model assumptions, articulate limitations, and, crucially, justify how these limitations do not compromise the model’s safe and effective application.
Learning from Past Failures to Prevent Recurrence
Engineering disasters like the Tacoma Narrows Bridge collapse can occur when engineers fail to recognize a model’s inherent assumptions and limitations. While engineering education often imparts model development and application, explicit focus on model limitations is frequently lacking. Enhancing students’ engineering judgment through targeted educational interventions is crucial to prevent future incidents akin to “Galloping Gertie.”