Thunderstorms over Indonesia, seen from the International Space Station
NASA Earth Observatory / International Space Station (ISS)
AI Weather Program Rivals Supercomputers in Forecast Accuracy
Scientists have developed an artificial intelligence (AI) weather program that reportedly achieves forecast accuracy comparable to conventional models requiring hours or days on supercomputers, but in just one second using a desktop computer.
Traditional Weather Forecasting Methods
Since the 1950s, weather forecasting has depended on physics-based models. These models utilize data from satellites, weather balloons, and stations to extrapolate weather patterns. This approach, known as numerical weather prediction (NWP), involves complex computations demanding substantial computational resources, often requiring powerful and costly supercomputers.
The Rise of AI in Weather Prediction
In recent years, researchers have increasingly explored artificial intelligence to enhance weather forecasting efficiency. For instance, Google researchers developed an AI tool to replace segments of intricate code within weather models, significantly reducing computational demands. DeepMind expanded on this by employing AI to generate complete forecasts. The European Centre for Medium-Range Weather Forecasts (ECMWF) has also adopted this trend, introducing the Artificial Intelligence Forecasting System.
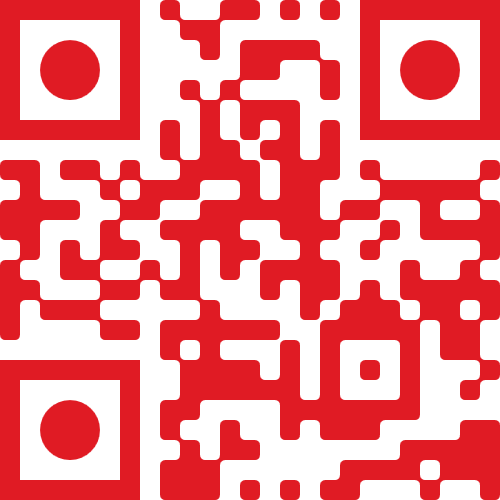
vCard.red is a free platform for creating a mobile-friendly digital business cards. You can easily create a vCard and generate a QR code for it, allowing others to scan and save your contact details instantly.
The platform allows you to display contact information, social media links, services, and products all in one shareable link. Optional features include appointment scheduling, WhatsApp-based storefronts, media galleries, and custom design options.
Aardvark Weather: A Comprehensive AI Model
However, previous integrations of AI in weather prediction primarily augmented traditional numerical methods, rather than fully replacing them. Richard Turner and his team at the University of Cambridge have developed a novel model aiming to overcome this limitation.
Addressing the Initialisation Gap
Turner explains that earlier AI implementations primarily focused on the forecasting stage, neglecting the “initialisation” phase. Initialisation involves collecting, refining, processing, and integrating data from global satellites, balloons, and weather stations into a structured grid – the foundation for any forecast. Turner notes, “Initialisation constitutes approximately half of the computational resources required.”
Their model, named Aardvark Weather, uniquely replaces both the forecasting and initialisation stages with AI. Remarkably, it achieves comparable forecast accuracy to contemporary NWP models while utilising only 10% of the input data, as detailed in their research.
Desktop Speed and Efficiency
Aardvark Weather can generate a complete forecast in roughly one second on a standard desktop computer. In contrast, traditional NWP forecasts can take hours or even days on powerful supercomputers.
Limitations of Grid Resolution
David Schultz, from the University of Manchester, points out limitations regarding Aardvark’s grid resolution. Aardvark employs a 1.5-degree grid, whereas the ECMWF’s ERA5 model uses a much finer 0.3-degree grid. This coarser resolution in Aardvark may limit its ability to accurately capture complex or unexpected weather phenomena.
Schultz cautions, “Unresolved factors could significantly impact forecast reliability. The model’s current scale may not adequately represent or resolve extreme weather events.”
AI Model Training and Reliance on Physics-Based Models
Turner counters that Aardvark may outperform certain existing models in detecting unusual events like cyclones. However, he acknowledges that AI models like Aardvark are trained using data from physics-based models. “Removing the training data derived from physics-based models and solely relying on observational data proves ineffective for training,” Turner states, referencing their attempts at developing a completely physics model-free approach.
Future Directions in Weather AI
Turner envisions the future of weather forecasting involving the development of increasingly precise physics-based models, which will then serve to train AI models for faster and more hardware-efficient output replication. Furthermore, some experts hold even more optimistic views on AI’s potential.
Potential for AI to Surpass Traditional Methods
Nikita Gourianov at the University of Oxford suggests that AI could eventually produce weather forecasts superior to NWP. He believes this can be achieved through AI models trained solely on observational and historical weather data, independently generating accurate predictions without relying on NWP. Gourianov emphasizes, “It’s a matter of scale and methodological ingenuity – cleverly inputting data and structuring the neural network.”